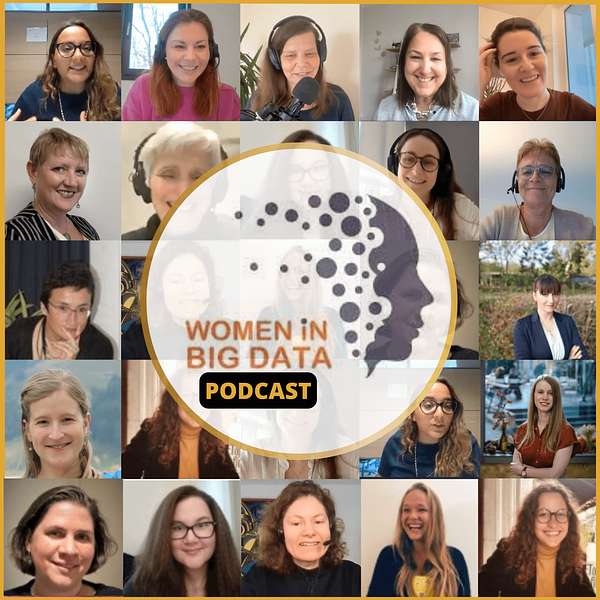
Women in Big Data Podcast: Career, Big Data & Analytics Insights
We connect, engage, and grow Women in Big Data by sharing their stories and experiences, while also unlocking their full potential through insights and advice from industry experts and thought leaders.
By doing so, we discover Career Insights and learn how to harness the power of Big Data and Analytics to stay ahead of the curve, drive innovation, and create a better future for all.
Women in Big Data Podcast: Career, Big Data & Analytics Insights
6. The Past, Present & Future of Bias in AI - A Talk With Karen De Sousa Pesse (Salesforce) & Anita Prinzie (Omina Technologies)
Listen, and get insights into The Past, Present & Future of Bias in AI in this talk with Karen De Sousa Pesse, Senior Executive at Salesforce & Anita Prinzie, Product & Marketing Manager at Omina Technologies.
Mentoring
Mentoring Program - Women in Big Data
Books
Invisible Women: Exposing Data Bias In A World Designed For Men (Caroline Criado-Perez)
Wij Robots (In Dutch by Lode Lauwaert)
Podcast
Visible Women (Caroline Criado-Perez)
Partnership
We are thankful to Digital Wallonia & Agence du Numérique for their partnership.
LinkedIn: Digital Wallonia
LinkedIn: Agence du Numérique
Hey There! Become a supporter by clicking the link above and help us create great Women In Big Data Content for listeners everywhere who want to learn about Career Insights and how to harness the power of Big Data and Analytics to stay ahead of the curve, drive innovation, and create a better future for all.
Mentoring Program - Women in Big Data
Mentoring is essential to success at every stage of a women’s career, both as a mentee and mentor. The many WiBD mentoring programs are open to WiBD members and cover opportunities for junior, mid-career, and senior women in technology. Not yet a member? No worries. By joining a mentoring program, you automatically become a WiBD member. Both membership and mentoring are free of charge.
Website: Women in Big Data Podcast
LinkedIn: Follow - Women in Big Data
LinkedIn: Follow - Women in Big Data Brussels
Contact us: datawomen@protonmail.com
Note: Podcast transcription edited to improve readability.
Desiree Timmermans 00:02
Hello, welcome to the Women in Big Data Brussels Podcast, where we talk about big data topics with diversity and inclusiveness in mind. We do this to inspire you and to connect, engage, grow, and champion the success of women in big data. The aim of this podcast is to reveal to you what you can do with big data, how organizations and societies use it, and the potential of big data to create a better future for everyone.
Karen de Sousa Pesse 00:31
AI in the past used to be the bias tool, and in fact, in the future is going to be the tool that prevents bias.
Desiree Timmermans 00:41
In this sixth episode, Valerie and I talk with Karen de Sousa Pesse, Senior Executive at Salesforce, and Anita Prinzie, Product and Marketing Manager at Omina Technologies, about Bias in AI. We cover the past, the present, and the future.
Let's start!
Desiree Timmermans 01:03
Welcome, Karen. Anita, thanks for joining Valerie and me to talk about Bias in AI. So Karen, can you tell us more about the history of bias in AI and where we stand today?
Karen de Sousa Pesse 01:14
Absolutely. Thanks for inviting me, by the way. We talk a lot about the AI bias and the bias in the technology that we are building. And it is interesting, for me personally at least, to understand where is this coming from? To build an AI model, you need data, right? You need data to build these models, and then you are going to do some correlations, and your models are going to be biased. And why is this data biased, then? And what are the implications for us?
Karen de Sousa Pesse 01:44
During World War II, roughly 6.7 million women had to go to the workforce because the men were not there anymore. They suddenly needed many people really fast, and the women were available. A large share of these women was working in very male-dominated jobs: constructing aircraft and assembling ammunition. In the manufacturing industry alone, they counted more than 3 million female factory workers between 1940 and 1944. But the thing is, as soon as wartime ended, most of these women were fired because a lot of these jobs were promised to the veterans once they came back. But just this moment alone was very important because women got a taste of what it is to, you know, work, have your own money, become independent, and so on. It created a movement of women that really wanted to work.
Karen de Sousa Pesse 02:42
During World War II, women were eventually doing most of the ballistics computing. When you think about that, in 1943, almost half of the people employed in the ICT sector were women. Where did it go wrong? Even though women were roughly 50% of the computer programmers, not many of them were promoted to leadership roles. The leadership remains male-dominated, not only in computing but also in other industries. The point is that leadership has historically been dominated by men. And the decisions that are being made are decisions that lack gender perspectives.
And even though women have been trying very hard to integrate into the job market, there were so many historical barriers to them. And then you reach the point of very few women in leadership; most of the decisions are being taken by men. And if everyone has their bias, a lot of these biases are going to be gender-related because a lot of the decisions are not being taken by women. So you see, all these events led to the point where we generated much biased data: a lot of data that lacked gender perspective and didn't account for differences between men and women. And once you get all this data, and then you train AI models - completely unaware of the bias that exists - you will be confronted by the bias.
Anita Prinzie 04:15
What you are referring to is historical bias. There is a certain bias and prejudice in society, which sinks into the data on which the models are trained. And I think one canonical example is the Amazon AI-enabled recruitment tool. Historically there have been more males in technical jobs than women. Also, the algorithm had another issue, which actually leads me to the second concept of representation bias. So it was an algorithm aimed at automating the recruitment of IT jobs at Amazon.
Also, there was a bias in the data because more females were quitting the job after a year than, for example, males. So by taking that time window of two years, there was already an implicit bias in the representation of the problem. So Amazon scrapped its AI-enabled recruitment tool because it clearly favored male applicants. Also, I think it is very important when we try to support decisions in a society that we really think about how will we represent the problem?
Anita Prinzie 05:23
A similar example of representing the problem properly occurs in the United States. So in the United States, they used an algorithm, and they might still use it to decide which patients should get extra medical care. The end goal was that they wanted to estimate who would meet the most extra care so that they would be treated in the hospital, and those with a lower probability of needing extra care would be treated out of the hospital.
Unfortunately, how did they represent this problem: they looked at not the need for extra medical care, but the target, in this case, was the future cost of care: how much people would spend on medical care. And when you think about this target, this outcome is not a race-neutral measure because it is proven that blacks with similar chronic conditions as white patients historically have spent much less on medical treatment because they go less often to a doctor than white patients. So, just by the way they represented the outcome measure, there was already much bias in the whole system. The AI had to be biased because it learns how much people spend going to the doctor. I think that's a very important lesson.
I think whenever you try to automate a certain decision with AI, it merits a lot of attention on how you will represent the problem and think about: could it be that this implicitly has some gender bias or ethnic bias, and so on?
Desiree Timmermans 07:00
Are there other things important if you want to fix the bias in AI?
Anita Prinzie 07:04
I think there are, broadly speaking, two things. On the one hand, many mathematical and analytical tools are available to analyze your data for existing historical bias. Obviously, you can try to avoid a representation bias, but there are many technical tools to detect bias based on the chosen group fairness metric. So the most common ones to be very concrete are disparate impact and equal opportunity. Professor Sandra Wachter clearly pinpointed, for example, European legal definition is matching very well, the equal opportunity. Also very important to know is that there are many potential definitions of group fairness. And why is that so important? Because it is your mathematical reflection of what a fair decision stands for.
Karen de Sousa Pesse 07:54
Yes, I completely agree with you. And in fact, one of the things that I tell people very often is that women are now getting highly educated on gender topics, but we lack men in the conversation. So, we need to start focusing on how we bring men as allies? How do we bring this conversation to them as well, so they are aware of what is happening? I really feel like now is the time in which we start bringing everyone into this conversation.
Valerie Zapico 08:23
I think the professors at the universities should also be giving some training about bias and how to manage this to be conscious that there is bias and that we have to keep that in mind.
Anita Prinzie 08:35
It is important that if you reflect on decisions and say, 'one group is not treated as fair as the other group,' you involve all parties and openly discuss what is happening. So to that extent, I agree definitely that we need to involve males in the process for sure. To some extent, they are probably already in the process. But it is also whether, or perhaps, looking into our ethical STEM because we do not every day reflect on our ethical standards. Certain policies are also active and have been there maybe for 20 years. I think many of these policies were devised a long time ago by mainly male leaders. And that is why they still reflect that kind of predominantly male perspective.
Desiree Timmermans 09:26
And how do you do that within Omina Technologies?
Anita Prinzie 09:29
We recruit in a very diverse way. So we do have people from different ethnic backgrounds. I think part of the solution is also still investing in STEM because we still see when you have a job opening that there are still more male candidates than female. The other thing we want to start doing is measuring the bias in your team. There is something like the implicit attitudes test, which tells you what kind of bias you have in your team. And based on the results of that test, you can decide, obviously, to first make sure that you communicate that you have a certain bias and try to overcome it within the existing team. But apparently, you can also use it to guide your future recruitment. So to make sure, if you have a certain gap in your attitudes - your attitudes might be a little bit biased - to recruit somebody that can bring in that perspective.
Karen de Sousa Pesse 10:28
Absolutely. I think not even bias but really unconscious bias. That is one of the biggest issues we have because I believe that the vast majority of the people who have these biases are taking these decisions simply because they don't know. And how do you know what you don't know? So we really need to look into our own bias overall and take it on an educational level. I really do wonder, and that is actually an actual point that I am taking from this podcast: if the universities are now educating their students about diversity, inclusion, AI, gender bias, any types of bias in AI?
Valerie Zapico 11:08
Karen, I have the chance to give a conference to reduce the bias in big data analytics and data science. It should be all along the master, the bachelor.
Karen de Sousa Pesse 11:19
The earliest you teach this, the best, right? So, I think this should even be taught in schools because what companies are doing now with all these diversity & inclusion policies is basically covering, putting a patch on the issue. So when I go to some events - and I talk to corporate people - and they discussing it amongst themselves, I say: look, the most important thing that you can do today for humanity about this topic is to go back to your children and educate them about what I just told you. Please bring it to your small children and educate them from a very early age that everyone has a bias, an unconscious bias, and what this can lead someone to do or think about.
Desiree Timmermans 12:02
So we already talked about the past and the current situation of bias in AI. I also would like to look at the future. For instance, Valerie, what do you think?
Valerie Zapico 12:13
For me, I would say mainly in Europe because we can speak about US and China, but it's totally different. In Europe, they define a tool, an assessment, that lets us check some cases and determine if the AI is trustworthy and ethical. This assessment should be more and more detailed in the next years for me, and maybe that would be a reference to be sure that we are all included in this topic, I would say.
Desiree Timmermans 12:44
So what you are saying is that it is a work in progress.
Valerie Zapico 12:46
When you develop an AI program, you need to maintain it. So you need to check it again and again to be sure that it is still trustworthy, ethical, and fair.
Desiree Timmermans 12:55
I understand. What do you think, Karen?
Karen de Sousa Pesse 12:58
So, AI in the past used to be the bias tool, and in fact, in the future is going to be the tool that prevents bias. Because if you take, for example, the cases of recruiting and hiring that became so popular, you can also build AI models that are going to make recruiting and hiring completely unbiased. For example, when you are interviewing someone, you always have this little bias, you know, if it is a person that comes from the same city as you and you speak the same dialect. And the AI will be able to be much more impartial about all the small biases that cloud our judgments. I really see AI is acting exactly the opposite, breaking out the bias and making fair decisions. AI is going to bring us clarity and insight into the future.
And the thought I wanted to close with is that AI is created by people. It is not artificial intelligence that is a bias; it is really our society. And AI is only augmenting this bias; it is only being a mirror. So I really see AI in the future as the mirror that shows us: hey, this is bias, this is wrong, and there is proof. So, that is where I see AI evolving towards.
Desiree Timmermans 14:13
Okay, thanks for that. And you, Anita, the future of AI. What does it look like?
Anita Prinzie 14:19
I think today, we mostly reason only on the data and the outcome of our standards in the past. So what if tomorrow, our AI is not only looking at historical data but also taking into account our future policies or future ethical standards? What I would like to stress is that you could define your ethical standards today and design your AI algorithm in such a way that it will meet those standards.
We need to have a paradigm shift from being reactive and testing post hoc whether the AI algorithm is biased towards gender towards a situation where we a priori decide what kind of behavior and what kind of standards we expect in terms of making decisions towards certain protected groups. For me, that is the biggest challenge for AI in the future. How can we combine data policies and then make decisions that meet those standards? I think it is important that the community thinks about how you can drive future decisions by not only looking at historical outcomes - which are the result of past policies - but also including the policies you would like to adhere to in the future.
Desiree Timmermans 15:37
I understand. So are you then also saying that we have to hold ourselves to higher standards? And that is something that we will see back in the algorithms?
Anita Prinzie 15:48
You need to make your algorithm aware of having certain standards. Now, we assume that the algorithm is aware of the standards we want to meet. If you don't optimize AI solutions beyond accuracy beyond interpretability, and you don't optimize them for your particular definition of fairness, you cannot expect that, magically, the AI solution will meet your ethical standards.
So we need to reflect on how we can, instead of the traditional objective functions of algorithms that mostly optimize accuracy, and sometimes they are optimizing already interpretability: how can you also include other measures of fairness, and so on? There are already some options in the literature to be completely transparent. There are other options as well. And that is what we are trying to look into. If you take, for example, the European AI Act, if you take non-discrimination acts, there are particular legal constraints. But obviously, every company has its own standards as well as society. So if you can reflect those mathematically, and you tell your algorithm: you have to create an AI solution that basically needs to adhere to the hard legal constraints but also to the more softer constraints of what your preferences are in terms of how the AI solution will make decisions towards women. That is something we need to look into: how can we build systems that account for this kind of objective, in addition to just optimizing for interpretability and accuracy?
Desiree Timmermans 17:24
That is clear. And if our listeners want to dive deep, do you have some resources that they can check out?
Karen de Sousa Pesse 17:31
I am going to recommend to you my all-time favorite book. And this book is really the one that I have read three or four times, and it is still on my bedside. It is: Invisible Woman - Exposing Data Bias In A World Designed For Men. It is by Caroline Criado-Perez, she is half Brazilian like me, and I am also very proud of that. The book is about how, historically, women were discriminated against and didn't have their perspectives heard. And how this all led to a huge data gap, a huge gender data gap. I really recommend everyone to read Invisible Women.
Desiree Timmermans 18:15
Thanks for that. Anita, is there a book or podcasts you recommend to our listeners to deep dive in?
Anita Prinzie 18:21
I would like to recommend a book by Professor Lode Lauwaert: Wij, robots. What I think is important in the book is the specification of the notion of justified and unjustified bias. That is a notion that is often overlooked: that some of the bias can be justified.
Desiree Timmermans 18:40
Well, thanks for this recommendation.
Karen de Sousa Pesse 18:43
Just one last thing. Because we are talking about different sources, this woman that wrote Invisible Woman also has a podcast - which is really nice to hear - and is called visible women.
Desiree Timmermans 18:56
Thanks for this addition.
Desiree Timmermans 18:57
Karin. Anita, Valerie, thank you very much. It was really good and a pleasure to talk with you.
Karen de Sousa Pesse 19:03
It was fantastic. Thank you very much.
Desiree Timmermans 19:06
Thanks for listening to the Women in Big Data Brussels' podcast. We appreciate it if you get in touch with us to provide your feedback or request to partner up and be a guest. You can contact us via data women@protonmail.com. You also find our contact details in the show notes.
Desiree Timmermans 19:29
Tune in next time!